Archive
Software Project Management Callback
Waterfall Project Management
“Waterfall” project management is a style of management best suited to the assembly and integration of proven processes and skills. In residential contracting, we work from plans, measure twice and cut once.
The materials, techniques, and skills required are known quantities. The quantities of steel, concrete, wiring, plumbing, and fixtures can be estimated from the plans, and established labor ratios can be applied to quantify the human component.
The challenge in these kinds of projects is wise management of the critical path, and the ability to adapt to early and late delivery, price surprises, material defects, resource scheduling conflicts, human error, and project changes.
In “waterfall”, the project manager is given an objective expressed as performance, time, and cost and must optimize these three variables while considering project risk.
Software project management is different.
By software development, I am not referring to routine low-code/no-code projects where workflows are tweaked, data is re-coded, and BI engine reports are created. Such endeavors today, especially with the assistance of AI have almost become predictable processes.
I am talking about software development where the development team must envision and create something new – much like a writer must create a fresh story in a series of entertaining books or scripts. They must imagine new usage cases, unintended consequences, and the product’s evolution along a future development roadmap.
A book author will often start with a conceptual sketch and prepare a rough outline of the written journey from start through exposition to conclusion. Details are filled in, usually not in the final chapter-by-chapter order, and drafts are refined until the story’s message is coherent, clear and impactful.
This kind of iterative development is a process of systematic discovery.
Iterative Development
The conceptual alternative to “waterfall” can generically be termed “iterative development,” and it is an approach that is decades old, and it predates computer technology and code creation, and has many applications outside of IT.
Fredrick Brooks, the well-known UNC computer scientist and thought leader, had some success with waterfall techniques when he redesigned the roles of a lean programming team. His original book, The Mythical Man-Month, is a classic of software development and gave us several guiding principles including: “Adding programmers to a late project will make it later.”
In subsequent editions of this book 20 years later, he explored the concept of iterative development. In simplest terms, it builds on the lean programming team model and adds iterative development constraints.
A development cycle is a short, relatively fixed time-period where an application is constructed and enhanced with only the functionality that the time budget allows. He calls this “growing a program.” This approach turns learning, discovery, and invention into a manageable process.
At each stage of development, the result is both a working product and a prototype for the next iteration. With this approach, nuances of a new language or strengths/limitations of a chosen framework of building-block functions can be explored, and adjustments can be made as necessary.
Project planning is developmental. Much like curriculum development for first, fifth, and 12th grade education, project planning has a vision, or outline, for what the product could look like at different stages of development. You can see that this lean style of iterative project management is “baked into” the DevOps and CI/CD methods and tools offered by AWS cloud services.
With either “waterfall” or “iterative” management, one wants control and desires to avoid the pitfall of the illusion of control. Project management skeptics who are aware of such illusions sometimes ask why plan at all?
I ask them to imagine productivity if we planned 100% of the time (the answer is none), and then I make the case that activity without performance-time-cost objectives will not deliver value. What is the value of the perfect wedding cake delivered a week after the wedding? What if the cake is cheap and on-time but looks and tastes awful?
Without a sense of where we are going, where we are, and an idea of how to get from here to there, the risk of failure is almost certain. A sweet spot of planning and control is somewhere in between no plan and an obsessive attempt at over-control. However, when we encounter those things we cannot control, we realize that plans must change; and that is okay.
An interesting X post had detailed criticisms of SCRUM and other recent academic attempts to build management layers on the thirty-year-old lean development framework of Dr. Brooks. The X writer did not reference The Mythical Man-Month, but the book certainly came to my mind when I read the online comments and replies.
On reflection, it also made me remember the advice: “…things I can control, the things I can’t. The wisdom to know the difference.”
© Operation Improvement Inc 2025. All rights reserved.
Dependencies and The Decision-Making Sequence
What is wrong with this management agreement?
Original: “The managing agency shall be paid 8% of gross revenues each month on or before the 25th.”
(Here is a hint: Look at the re-worded agreement, clarifying understanding and remove any ambiguity.)
Revised: “The managing agency shall be paid 8% of the preceding month’s gross revenues each month on or before the 25th.”
There are two activities implied in each of these two statements. (1) One month’s gross revenues shall be totaled. (2) Eight percent of the total shall be calculated and paid.
A dependency diagram would make this clear. Calculating a (prior) month’s gross income comes first, and calculation of a management fee is a dependency, but it is not simply a dependency in time!
Most people assume that dependency diagrams are flow charts or time sequence charts. They are NOT!.
Dependency Diagrams are best described as knowledge and product dependency Diagrams
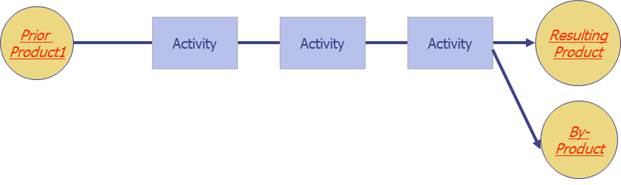
Transient Products
Each process activity box in a dependency diagram could be drawn on a separate page. A resulting product could be shown for each process step, and that product could be shown as the key ingredient, or incoming product, to a subsequent step.
Instead, these transient products are typically not shown, unless a deeper level of detail is really required to understand the work. Process steps are connected one to the next, and these transient products are implied in the diagram.
In the management agreement example, the calculation of gross revenues produces a product: a dollar sum. That transient product (the dollar sum) is the input to the calculation of a percent management fee.
If a transient product is flawed, all subsequent process steps are voided!
The dependency diagram for calculating management fees reminds us that a correct percentage calculation depends on a correct sum of the previous monthly revenue. If prior months revenue calculations are incorrect, all downstream calculations are null and void.!
It is an understandable mistake to think that dependency diagrams show time sequence, but the deeper interpretation is that a bookkeeper must know the monthly revenue sum before a correct management fee can be calculated. It is in this sense that dependencies illustrate knowledge dependencies.
Dependencies In Project Management
Dependency diagrams are a essential tool in process management, but the concept of knowledge dependencies may be clearer if we draw a couple of examples from the other branch of tactical management: Project Management.
Knowledge dependencies may be clearer if we take a look at PERT chart techniques in project task scheduling.
In a systematic scheduling of tasks, task relationships are typically described as predecessor and successor tasks. Tasks are related in one of four ways: Finish-Start (FS), Start-Start (SS), Start-Finish (SF), and Finish-Finish (FF). In addition, a gap or overlap is specified as lead or lag time.
Novice schedulers just “know” that Task A (Build Forms) should happen on Monday and Tuesday, and Task B (Pour Concrete) should follow on Wednesday and Thursday.
If they use a software package, they fiddle with task dependencies and leads and lags until the two tasks appear on the calendar where they intended. This is not how these powerful tools were meant to be used.
The concrete contractors, let us say, are our most difficult to schedule workers. They have promised to bring concrete to our work site on Wednesday. That is the predecessor task. It is a bit of certainty that the rest of the schedule builds on.
Based on the knowledge that the concrete will be delivered on Wednesday, we establish a relationship with the successor task. We calculate that the building of forms to hold wet concrete must be finished before concrete is delivered on Wednesday. That is a Start-Finish relationship.
Since construction of footings is expected to be a two-day process, we determine a starting date for that task by the powerful mathematical technique known as subtraction. We work backwards on the calendar to find the Monday starting date of Task A.
The Gantt chart shows the time sequence: A precedes B. But the PERT chart preserves the knowledge dependency relationship.
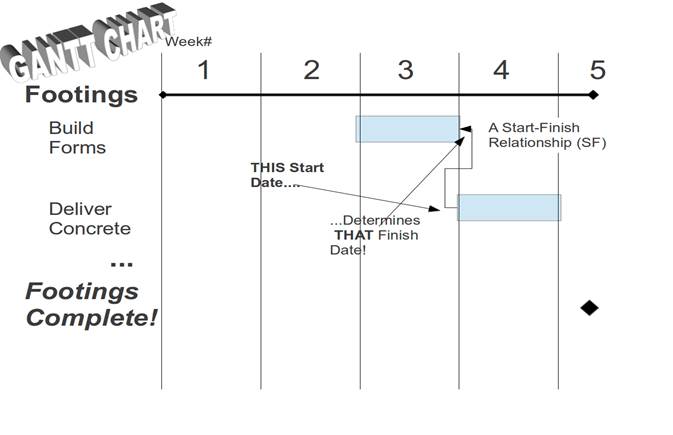
On the PERT chart view, Task B (Concrete) comes first, and Task A (Forms) follows. This is because we know with some degree of certainty when concrete will be delivered, and we are planning the other tasks accordingly.
We plan because there will be change. Things will not always go according to plan. If the concrete contractors reschedule, the PERT chart and the Start-Finish dependencies in our scheduling plan remind us which tasks must be rescheduled.
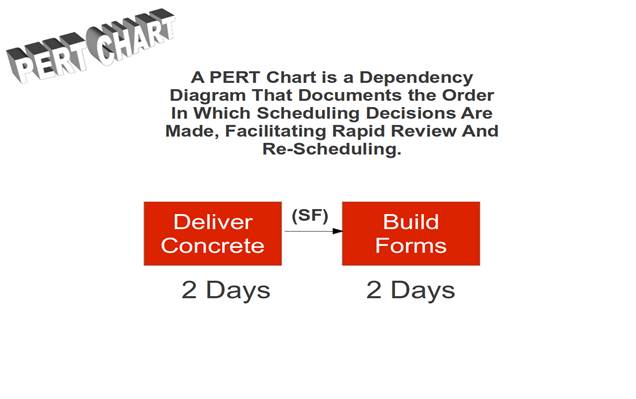
In process and project management, the tactical manager’s “go-to”move is re-deployment of resources in order to accommodate change, substitute equipment and methods, and in some cases – shorten the duration of resource-driven tasks.
The dependency diagram retains a record of causality in our decision-making. “Why did we schedule form construction when we did? Because the concrete contractors go on the schedule first, and other tasks are scheduled and rescheduled accordingly.”
Critical Path
Dependencies are used sparingly in project scheduling to represent real constraints. In our example, the difficulty of scheduling concrete had an impact on at least one other task.
A prepared bed for concrete complete with forms is something that may be perishable. Perhaps we do not want that work completed and left exposed to the elements for too long before concrete is delivered. There is a definite reason to incorporate this dependency in scheduling.
Other tasks are scheduled later by analyzing available resources. The limitation of workers and equipment keeps us from tackling every task simultaneously, and a project manager will work that out in a second phase of scheduling.
For now, these tasks that are independent are left on the schedule to start ASAP (as soon as possible). Resource limitations will cause some of these to be delayed.
At this early stage of scheduling, something called a critical path emerges. It is possible to identify a sequence of tasks that determine the minimum total duration of a project.
If there are no dependencies in a project and everything is scheduled ASAP, the critical path is simply the time it takes to complete the longest task.
When product and knowledge dependencies are identified and incorporated into the schedule, a realistic timeline of the project begins to take shape.
Novice misuse of scheduling software and dependency-based scheduling often results in everything being linked into a too long critical path. This creates unrealistically long estimates of the total project duration and leads the manager to think their only means of improvement is more resources.
Proper and sparing use of dependencies and ASAP scheduling gives the tactical manager options. They can choose where and when to deploy and redeploy resources. They can lengthen non-critical tasks and shorten tasks on the critical path.
When things change, when things do not go according to plan, the most powerful tool in the tactical manager’s kit is the power to redeploy resources. A good plan establishes contingencies in advance.
The Factor Table
Dependency diagrams are not flow charts. Particularly in process management, they represent a planning of work that proceeds from certainty to certainty, from correct product to correct product.
How, then, can such a simple method for diagramming processes be made flexible enough to accommodate variability and unknowns?
Many are tempted to turn dependency diagrams into flow charts. They want to introduce decision box symbols and branching and looping lines and arrows. This is not only unnecessary; it is a mistake that will have a negative impact on the ability of a tactical team to work confidently, correctly, and consistently.
In another article, we apply dependency diagramming to work in call centers. In that call center example, our process is to greet, identify the caller, and then match the call request. Callers can call about a multitude of things.
Even if we factor the types of calls into a smaller and manageable number, how then does the manager maintain a sense of orderly correctness with a simple dependency diagram? If we decree that there are a dozen different types of calls, do we make a dozen dependency diagrams to plan and describe each process variant?
The answer is no, and the solution is something called factor tables. A discussion of factor tables deserves its own article in this series. Once mastered, you will never be tempted to turn dependency diagrams into flow charts.
© Operation Improvement Inc 2025. All rights reserved.
Managing Variability
Shoes in the shoe store were just not selling. After a careful survey of available shoe sizes (6, 8, 10, and size 12) and customer feet (7, 9, and size 11), we happily conclude that the average shoe fits the average foot. What did we miss? Variability.
Variability
The shoe store has some control over the variability in shoe sizes when orders are placed, but tactical management of variability is much more subtle. There are things that you can control and things that you can’t. In project and process management, the inability to tell the difference makes things worse and can even end in failure.
There is an old and silly joke about the man who tells the doctor, “It hurts when I poke my finger in my eye.” The doctor, of course, says, “Well, stop doing that!” Learn how to properly manage variability.
It is often, but not always, easy to manage known causes of variation. In one copper coil winding and insertion operation, our studies revealed a one percent chance of coil failure when current was applied.
Copper coil windings varied in how tightly packed the coils were. Some were wound a little loose and some a little tighter. On average, the tightly packed copper bundle was a perfect fit into the intended machine slot.
The loose coils were problematic. About one percent had to be forced into position with the only tool available to the operator, a screwdriver! Aha! The cause of defective coils! (Details of that journey are in another article.)
The problem could have been solved far upstream by a product re-design or by re-engineering the coil insertion process, but our simple solution to eliminate this defect was tactical management of variability.
No Silver Bullet
“Surely,” many managers think, “there is a product, maybe a tool, a machine, maybe software… something I can buy that will solve my variability problems for me.”
It is axiomatic that one needs tools suited to the task at hand; but while tools can be helpful, there is only one alternative to learning about how to measure and manage variability. The alternative is to suffer through it.
In service industries, many try to punt this issue into the Information Technology department. In call centers/contact centers, staffing never seems to match demand variability, so fortunes are spent on scheduling software and overly complex routing and load-leveling systems.
The common result is that calls are dispatched and routed in an inscrutable and unmanageable manner. Service quality suffers because calls are not relayed to the right people. (“But we bought skill-based routing!”) Caller wait times still have unacceptable peaks and valleys.
In manufacturing, parts assemblers given average-sized cylinders to be inserted in average-sized openings must fiddle with stacks of slightly under-sized and slightly over-sized “within specifications” parts. They then wonder why items that meet tolerances won’t fit together.
Without a proper understanding of variation, managers direct purchasing to tighten the tolerances and buy more expensive components, raising the cost basis of the product they want to sell.
Be Careful What You Ask For…
You may get it! One cannot apply brute force; one cannot decree that workers stop producing results that have variability. It does not work and only makes things worse. (In his Walkabout © series of books, James Abbott calls this mistake “Forced Capability”; a violation of the principle of Division of Labor in decision-making.)
One tactical manager tried to eliminate variability by decree. He told his three shift managers, “Do whatever it takes.” One shift manager performed measurably worse after the order. We called him the Good Trooper because of his story.
“Of course I’ll do what the boss said. I was in the army. I learned that when the boss says jump, you ask how high.”
The other supervisors performed as they did before the manager’s new rule. They said,
“He doesn’t understand what we can and can’t control down here, and he won’t listen. I guess whether or not I keep my job depends on chance.”
“There is a chance my variability numbers will be bad, but there is also a chance they will be good. I’ll take the credit if they are good and risk the consequence if they are bad. I know I’ll only make things worse if I don’t leave what is currently working alone.”
Manage or Re-Design
An architectural re-design of a process and re-engineering with and capital outlay (new equipment/automation) is a strategic decision. Tactical managers must be masters of variability regardless of the technology and tools employed. New equipment lets an operation make scrap faster!
Tactical management of variability is straightforward and methodical and requires five things:
- Targets. You must establish and oversee a correct operation based on what you presently know. This includes contingency plans to re-deploy resources when conditions change. (“If it rains, we will need umbrellas.”)
- Consistency. You must run that operation as consistently as possible.
- Fresh Data. You must measure and monitor variability in real time. Today’s data must be available for today’s decision-making, and not just at the end of the month.
- New Knowledge. You must learn new things – details about your operation.
- Managed Change. You must incorporate that new knowledge into a new baseline plan of correct process operations.
New Knowledge
Knowledge is power. The manager who has ten years of experience has an advantage over the one who has one year of experience repeatedly over ten years.
You can measure and report variability with statistical tools such as standard deviation and coefficient of variation, but your progress in the management of variability is measured by assessing your inventory of knowledge!
An apprentice carpenter may start with a basic inventory of three or four ideas: the hammer, the nail, the pointy end of the nail goes into the wood, and the desired flush finished result.
The skilled carpenter will know about knots in wood, the behavior of laminate materials, the risk of splitting thin strips of wood along the grain, and more.
Similarly, the experienced tactical manager should have a larger inventory of knowledge after accumulating time on the job. If you can master the skill of managing variability, you may very well accumulate your ten years of experience in a year, instead of the other way around!
Acting on New Knowledge
Some of the things you will learn will represent opportunities and some will represent risk. Some will be manageable and some will not.
Some will require new contingency plans so opportunities can be seized and not lost. Some contingencies may be provisioned to mitigate risk. Some will require a strategic response that will be beyond the scope of a tactical decision.
The important thing is to maintain a mental readiness, an awareness of the difference between the things you can control and those things that you cannot.
In another article in this series on causal analysis, we portrayed cause-effect and root-cause analysis as a potentially infinite chain of events with a decision fork in the alternatives where a human being has made a choice.
One decision fork in the analysis pursues the nature of things as far as we can until we rest our case with this conclusion: “And that’s just the way things are.”
The other decision fork terminates in human choices and actions. Of course, our past choices and actions become a given that no power in the universe can change. They are as indisputable as the fact that nails are pointy and will deflate a tire due to “the way those particular nails are”.
But we can shift our perspective on past human events and submit them to judgment and review. Why did we decide? Why did we choose? What would the outcome have been had we acted differently? Will we have the knowledge, when and where we need it, the next time a similar decision is made?
These are the questions we must ask if we are to incorporate and use new knowledge. These are the questions that highlight what, in the future, we may be able to manage and control.
© Operation Improvement Inc 2025. All rights reserved.
Strategy Versus Tactics
Dilbert and His Boss
There are two sides to an old argument, and I have heard both many times and in many circumstances.
The debate usually begins like this: Dilbert, who is a salesman, agent, operator, mechanic, accountant, engineer, programmer, or some other front-line soldier of business, puts the discussion in play.
“What a disaster in the making! Our new boss has no clue about the details of this business. No doubt they teach them in school that managers need not know the business to run it. This happened at the last place I worked, and they closed in less than a year. Better get your resumes ready!”
The other side of the coin is usually argued by a generalist manager who, by their protests, identifies themself as the boss that Dilbert is talking about. They want to defend their contribution to a company’s success.
“Well, I was hired to turn around XYZ, Inc., and I did it in less than a year! I reorganized (or reprioritized, rescheduled, relocated, re-incentivized) the organization with retreats, goals, Monday staff meetings, MBO, metrics. I don’t have to know what I am measuring to interpret the data. There is a place for the professional manager. Why, just look what happens to a company when they put an accountant, engineer, or a salesman in charge!”
We have all seen situations or heard stories that would seem to support both positions. This leads us to think that there might be an underlying condition, a hidden “if” that tilts a case study to one position or the other.
What Is It About the Organization That Needs Fixing?
In some businesses, operational flaws are minor, and the organization needs a cohesive vision and strategy. Such a business has well-designed processes and a tactical workforce that understands the business product.
Without direction, the best tactical teams often lack focus. A new manager may remedy this problem without spending a lot of time deep in the nuts and bolts of operations. The perfect analogy is a parked car with no place to go, its finely tuned engine running, waiting for a driver and destination.
Competent tacticians who are quite comfortable with tactical decisions are often paralyzed by strategic choices. When an issue is purely tactical, a T ledger can be made of pros and cons, or a clever programmer can code a decision tree. Even if some of the inputs are probabilistic (e.g., 40% chance of project delay due to weather), it is still possible to reduce the issue to a data-driven solution.
When tacticians are confronted with choices that require weighing values and qualitative risks or picking one of three alternatives, many tacticians find themselves in a Hamlet loop. They will debate the “To be or not to be” of the issue endlessly and without resolution. After exhausting everyone with all of their reasons for an initiative, they can return from a break with a hundred and one reasons against, suddenly opposing the position they just held before lunch.
One successful turnaround manager said, “My new staff had fourteen different visions of the company mission.” His success depended on getting the organization to focus on one vision, and not upon learning the details of each technician’s job.
But what if the organization is not a finely maintained and tuned race car looking for a driver? To continue the analogy, what if the car has not been maintained? What if parts have been removed and not replaced because they were too much trouble? How do you make an operation right, and then make it better?
Eventually, Management Needs to Get into the Details
I’m wary of the turnaround experts who brag that they can always “move the numbers” without knowing the operation. Many organizations in trouble have problems that go deeper than motivation, vision, and focus.
Sometimes managers fool themselves. They think improvements have been made, and they haven’t. A problem seems to disappear only to pop up somewhere else.
I know (and can uncover) most of the tricks to transfer costs from the business to the customer or to the employee, from the P&L to the balance sheet, from one department or plant to another, and from the short term to the long term. Such zero-sum changes to a business do not improve the operation and usually cause harm.
A further complication is that many traditional performance metrics are lagging indicators and can temporarily move in the wrong direction during periods of transition. I have two real-life cases that I have used in training classes where operations service level statistics temporarily became better as customer service got worse.
Managers who do not understand their business processes are terrified of a transition when the metrics are providing misleading feedback, and they often say, “I’m scared to run the business right!”
Eventually, all managers must develop an understanding of the details of their business operation. Those managers fortunate enough to inherit a capable tactical organization will eventually need to turn their attention to sustainment. Those who inherit an operation with incapable processes need details as a prerequisite to focus and vision.
As I have said in our Management Philosophy, “You need not be a surgeon to discuss brain surgery, but you should at least be able to define brain and surgery. If it is true that you can’t effectively manage without measuring, you surely can’t manage what you cannot define.”
© Operation Improvement Inc 2025. All rights reserved.